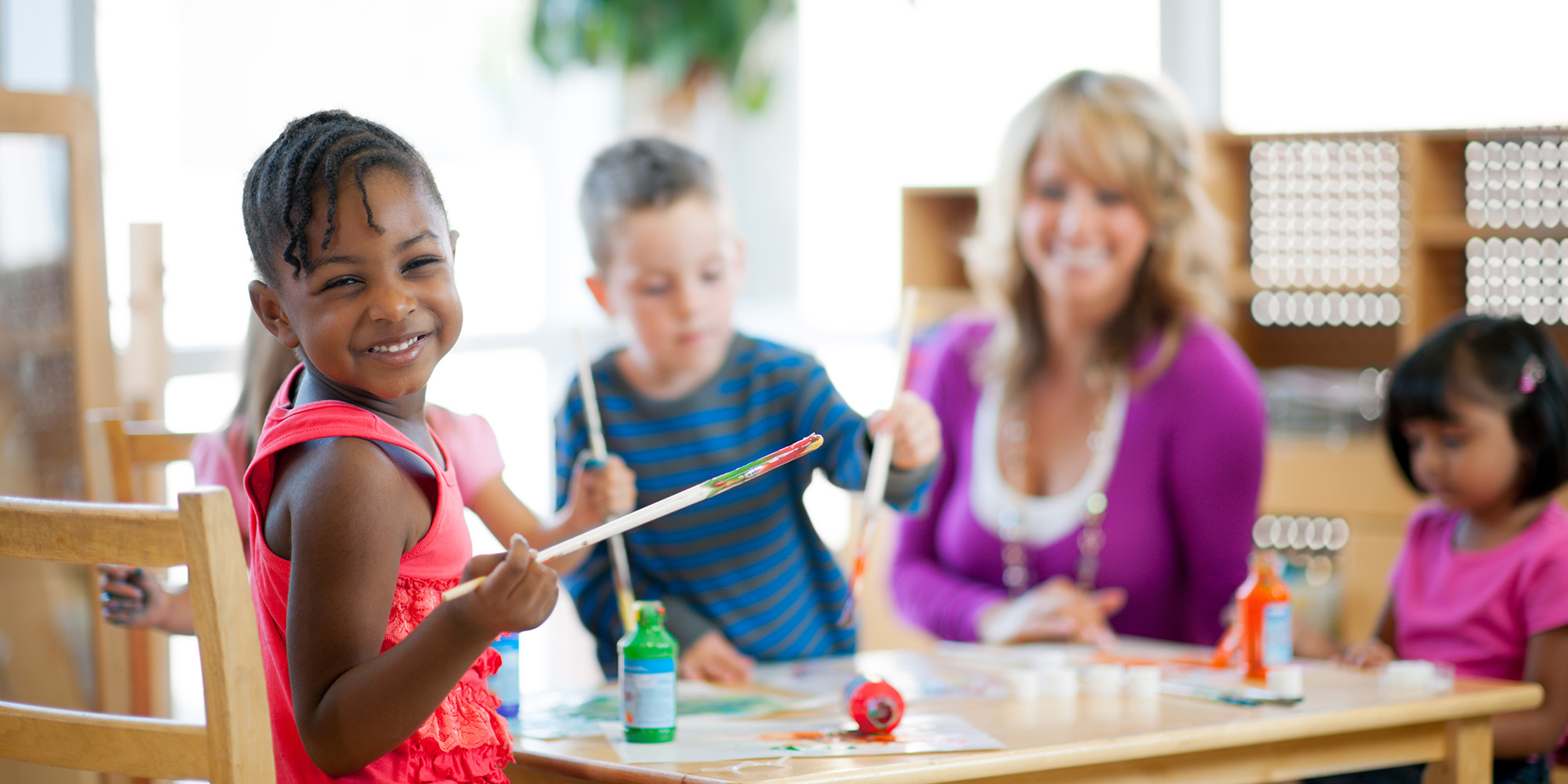
Investing in the Future of Early Childhood Education: Measuring Early Childhood Care Quality at Scale
The ability to measure the quality of Early Childhood Education (ECE) care at a large scale continues to challenge educators, researchers, and families in the U.S. and around the world. This provides the catalyst for our initiative—one that brings together a skilled, multi-disciplinary team focused on utilizing real-time video streams, computer vision, and automated scoring techniques to create an effective, scalable approach to evaluate the quality of care in ECE in a continuous, cost-effective, and scalable way. The result of this work is a game-changer—revolutionizing the future of our children’s development in ways that have never been achieved before.
The Need
The lifelong success of children depends heavily on the quality of education received during the earliest stages of life. The young child’s experiences crucially impact cognitive and socio-emotional development, making quality assurance a necessary facet of public investment in ECE. Current measures, based on infrequent, short-term observations (e.g., 3 hours every 2 years), often fail to capture an adequate picture of the ECE environment, leading to unreliable assessments that, if more accurate, could help ECE teachers constantly improve instruction for each child. Worse, the lack of trusted measures and outcomes also hinders policymakers in guiding future investment in ECE. At a time when philanthropic and government investments are most needed, the ability to find a solution to this problem is key.
The Solution
Our proposal bridges the evaluation gap by employing video streams, providing rich, continuous observations across the school year. To accomplish this, we’ve partnered with Class Proxima, a technology company specializing in early childhood, and WatchMeGrow, the leading U.S. supplier of childcare camera systems. With potential access to video data from 3,000 schools in WatchMeGrow’s network and Class Proxima’s capability for data collection and analysis using computer vision and AI, we’re ready to make a substantial leap in evaluating ECE quality to help teachers improve instruction and child outcomes.
The Impact
By successfully implementing this project, we will:
- Unveil a novel understanding of quality in early childhood education, generating large-scale, reliable observation data for the first time.
- Validate an economical approach that can be scaled across all ECE classrooms, transforming traditional quality control methods and significantly reducing costs.
- Provide anonymized benchmark datasets to assist in effective policymaking.
- Arm the ECE research community with groundbreaking tools, redefining their work and data collection processes.
- Ultimately, make a profound and lasting contribution to humanity’s most precious investment: our children’s development.
Project Team
We are an interdisciplinary team across multiple institutions. While science is at the forefront we also recognize the critical importance of diversity and expertise from educators working directly with children and families. We welcome recommendations for committed individuals to join us on this journey.
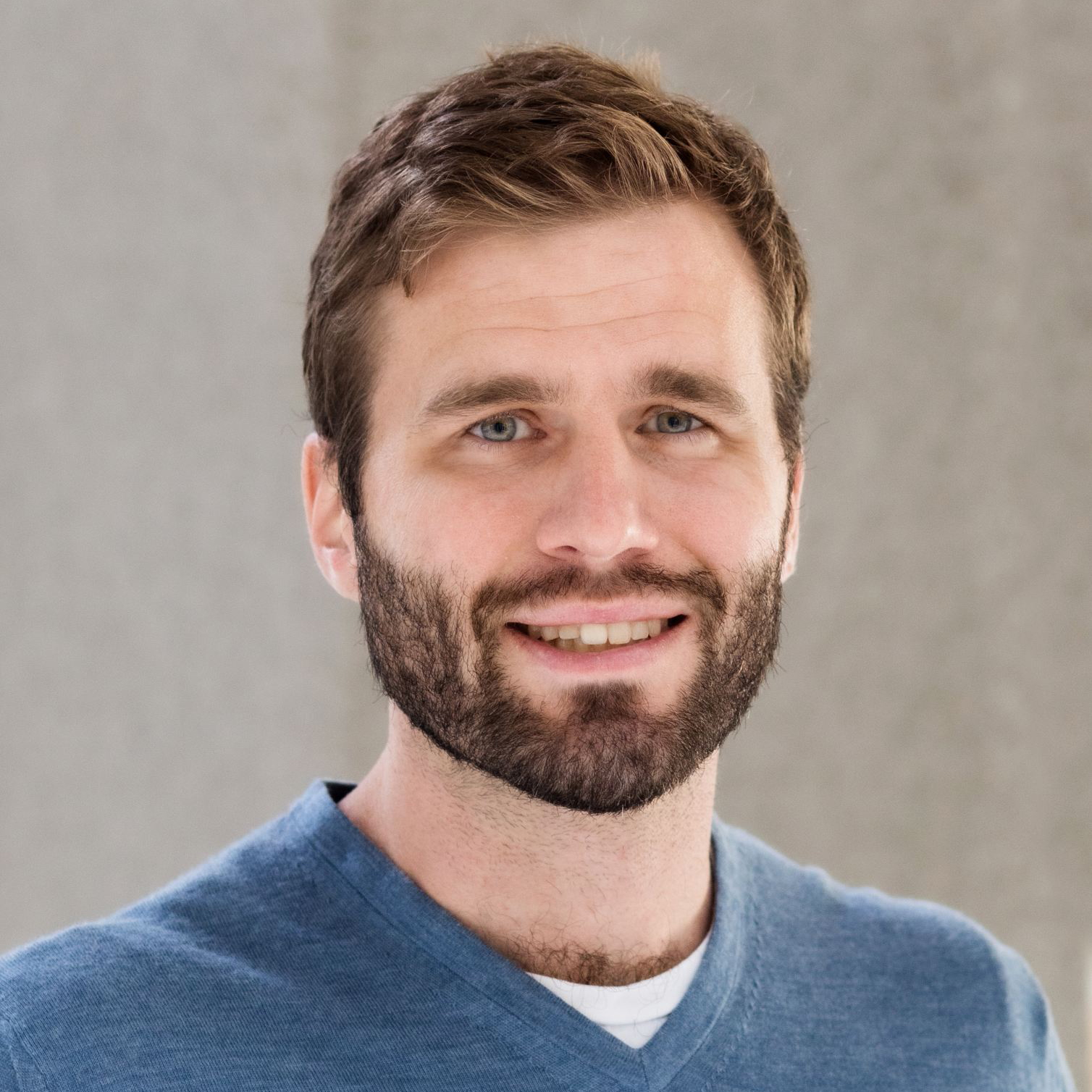
Drew Bailey
University of California, Irvine
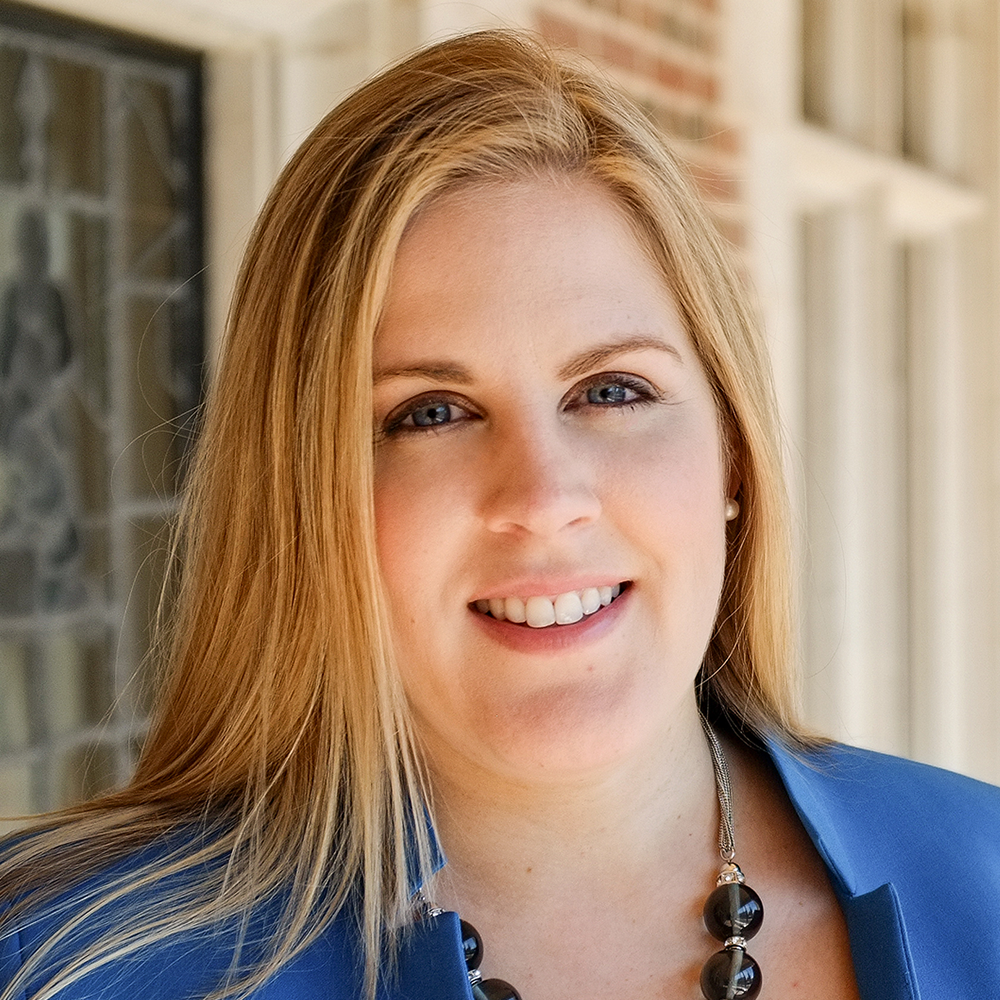
Alison Baulos
The University of Chicago
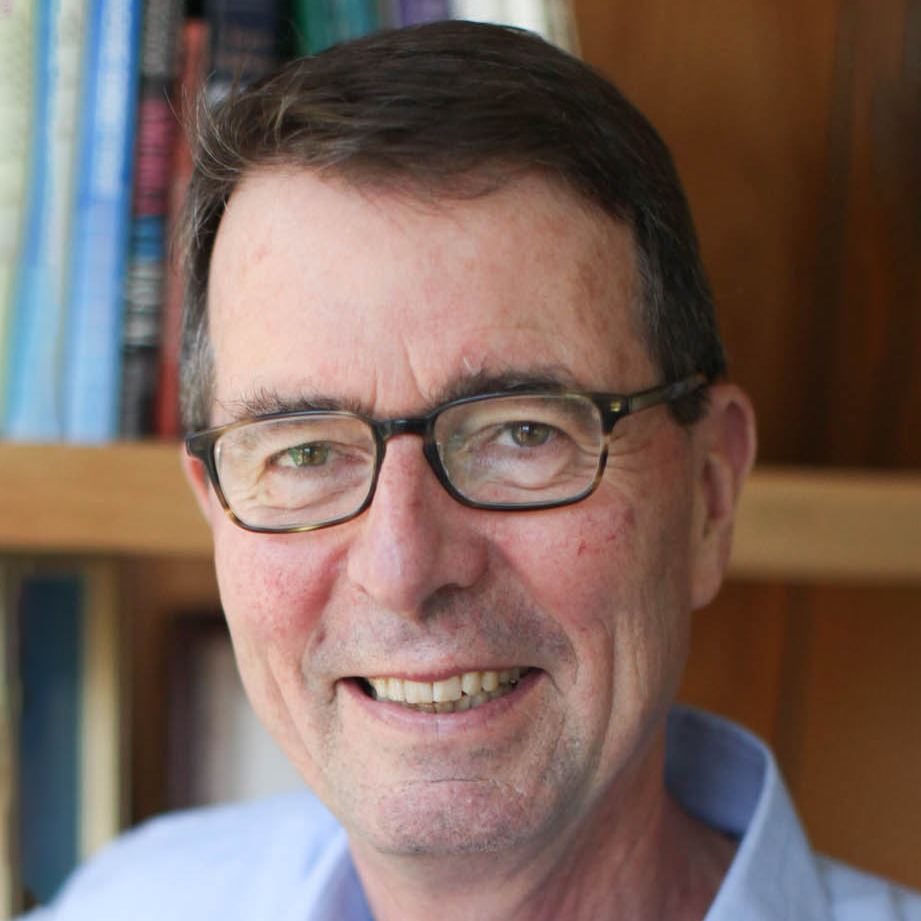
Greg Duncan
University of California, Irvine
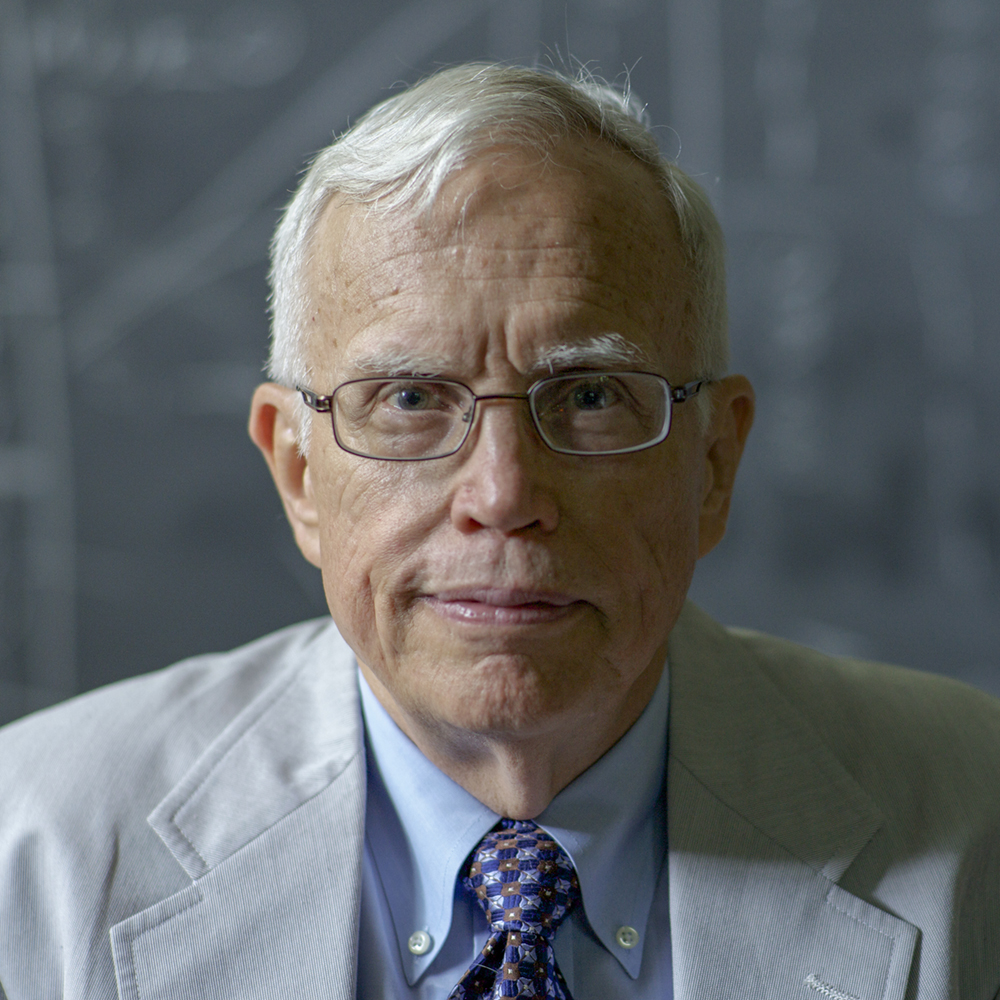
James Heckman
The University of Chicago
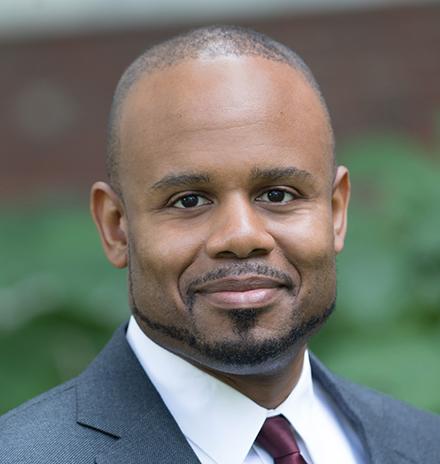
Edward McFowland
Harvard Business School
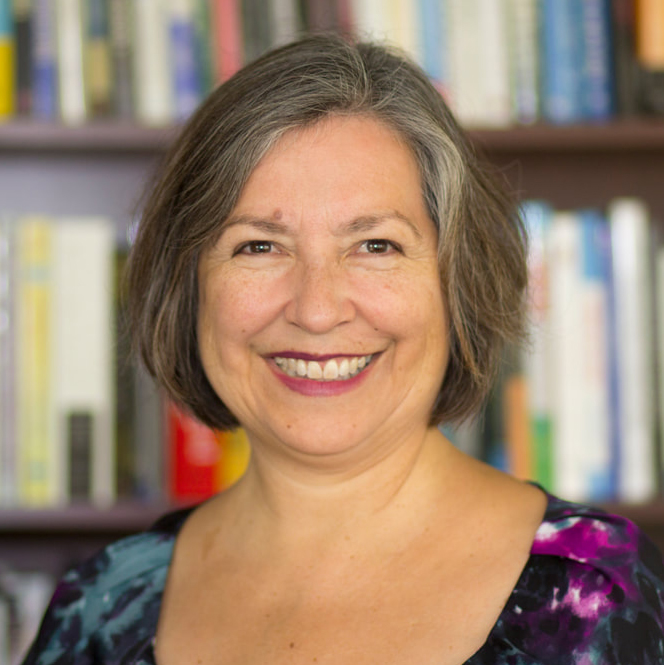
Elizabeth D. Peña
University of California, Irvine
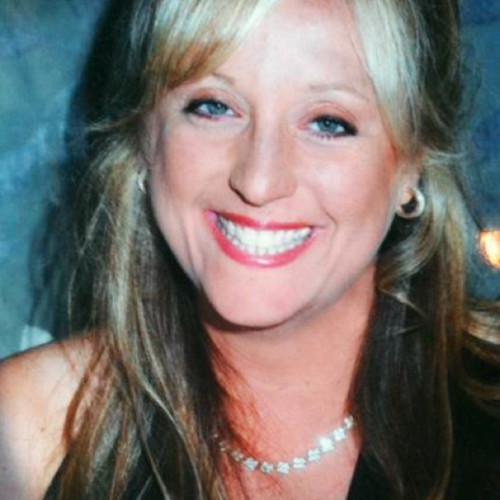
Aleisha Sheridan
4C Indiana
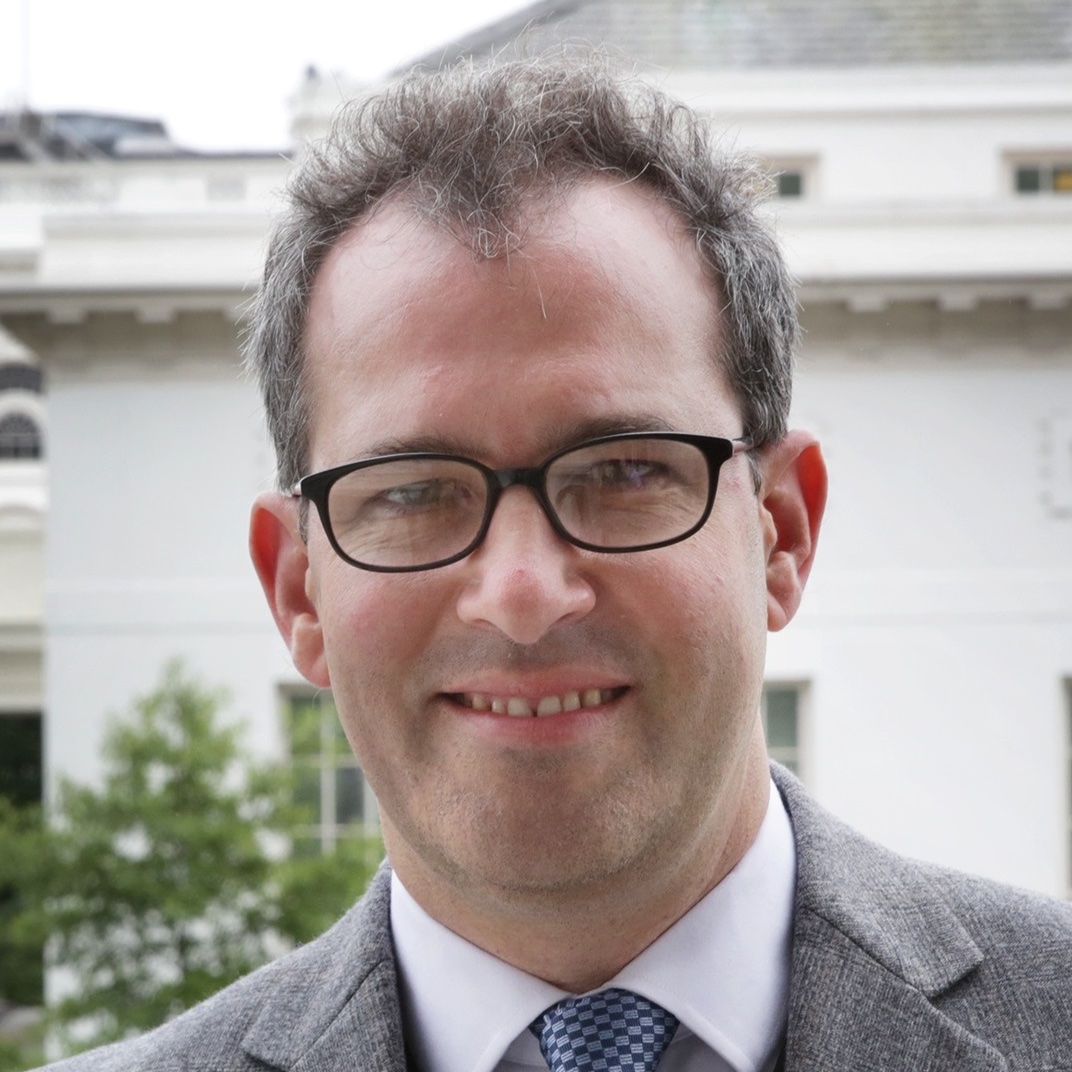
Aaron Sojourner
W. E. Upjohn Institute
Funders
This project is seeded through the Center for the Economics of Human Development and we are currently seeking funders